Florentin Hildebrandt
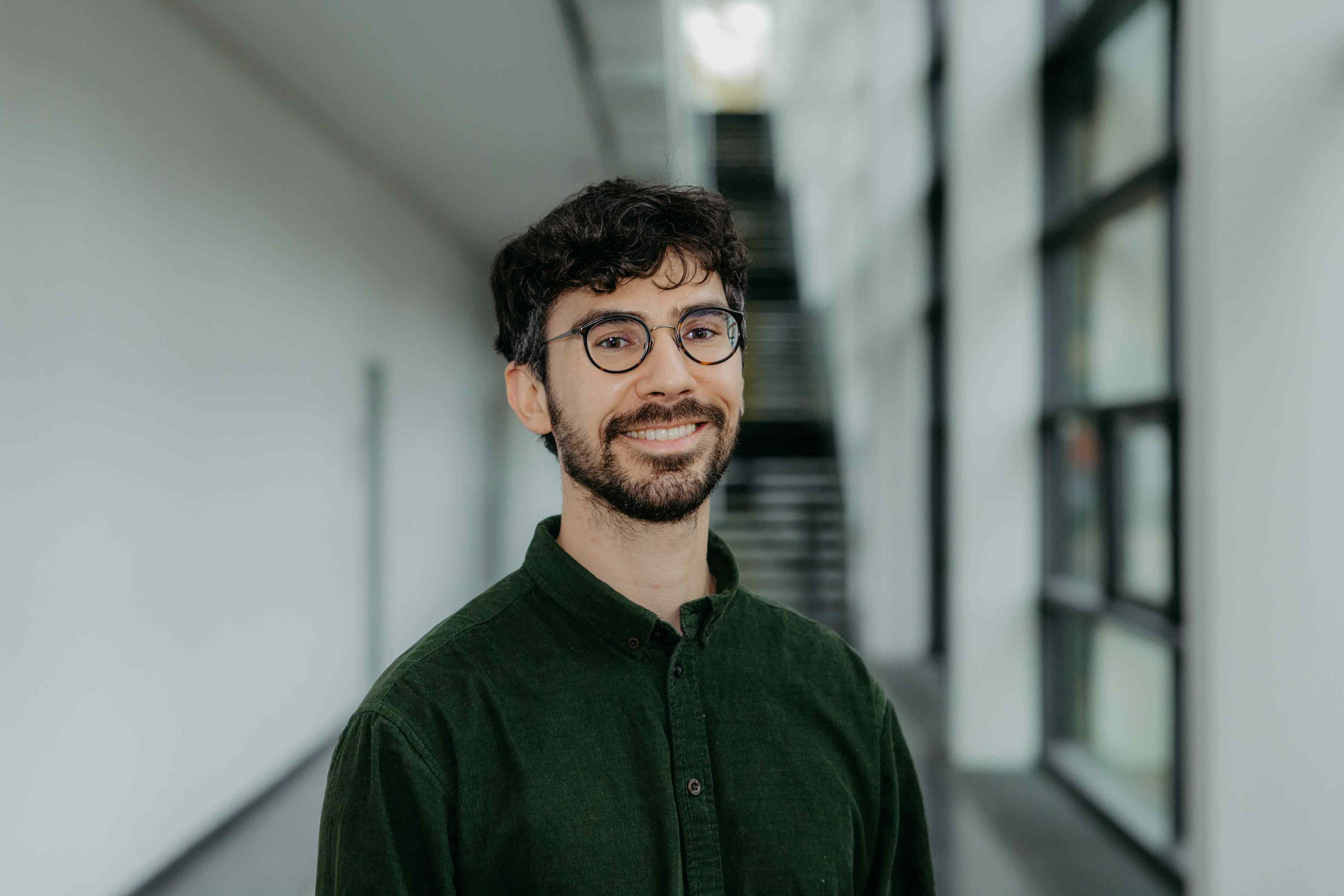
M.Sc. Florentin Hildebrandt
Lehrstuhl BWL, insb. Management Science
03/2023 - 06/2023 |
Visiting researcher, Dipartimento di Ingegneria dell'Informazione, Università di Padova | |
Since 10/2021 |
Research associate, Chair of Management Science, Otto-von-Guericke-Universität Magdeburg | |
03/2020 - 10/2021 |
Research associate, Decision Support group, Technische Universität Braunschweig |
|
10/2017 - 02/2020 |
Master of Science in Mathematics, Georg-August-Universität Göttingen |
|
03/2019 - 03/2020 |
Student worker, BESSY II, Helmholtz-Zentrum Berlin |
|
10/2018 - 03/2019 |
Intern, 4flow Management, 4flow |
|
10/2017 - 10/2018 |
Student worker, AG Optimierung, Georg-August-Universität Göttingen |
|
10/2013 - 07/2017 |
Bachelor of Science in Mathematics, Georg-August-Universität Göttingen |
2025
Peer-reviewed journal article
The restaurant meal delivery problem with ghost kitchens
Neria, Gal; Hildebrandt, Florentin D.; Tzur, Michal; Ulmer, Marlin W.
In: Transportation science - Hanover, Md. : INFORMS, Bd. 59 (2025), Heft 2, S. 433-450
Learning state-dependent policy parametrizations for dynamic technician routing with rework
Stein, Jonas; Hildebrandt, Florentin D.; Ulmer, Marlin W.; Thomas, Barrett W.
In: Transportation science - Hanover, Md. : INFORMS . - 2025, insges. 19 S.
Integrated fleet and demand control for on-demand meal delivery platforms
Hildebrandt, Florentin D.; Lesjak, Žiga; Strauss, Arne K.; Ulmer, Marlin W.
In: Management science - Hanover, Md. : INFORMS . - 2025, insges. 23 S.
2024
Book chapter
Tourenplanung
Ackva, Charlotte; Fassnacht, Lukas; Henninger, Steffen; Hildebrandt, Florentin; Spühler, Felix
In: Radlogistik - Grundlagen zu Logistik und Wirtschaftsverkehr mit Lasten- und Transporträdern - Wiesbaden : Springer Fachmedien Wiesbaden ; Assmann, Tom *1989-* . - 2024, S. 197-212
Standortplanung
Ackva, Charlotte; Fassnacht, Lukas; Hildebrandt, Florentin; Spühler, Felix
In: Radlogistik - Grundlagen zu Logistik und Wirtschaftsverkehr mit Lasten- und Transporträdern - Wiesbaden : Springer Fachmedien Wiesbaden ; Assmann, Tom *1989-* . - 2024, S. 177-195
2023
Peer-reviewed journal article
Opportunities for reinforcement learning in stochastic dynamic vehicle routing
Hildebrandt, Florentin D.; Thomas, Barrett W.; Ulmer, Marlin Wolf
In: Computers & operations research - Amsterdam [u.a.] : Elsevier, Bd. 150 (2023), Artikel 106071
Non-peer-reviewed journal article
Reinforcement learning variants for stochastic dynamic combinatorial optimization problems in transportation
Hildebrandt, Florentin D.; Bode, Alexander; Ulmer, Marlin Wolf; Mattfeld, Dirk C.
In: Magdeburg: Otto-von-Guericke-Universität Magdeburg: Fakultät für Wirtschaftswissenschaft, 2023, 1 Online-Ressource (38 Seiten, 0,82 MB) - (Working paper series; Otto von Guericke Universität Magdeburg, Faculty of Economics and Management; 2023, no. 06)
2021
Peer-reviewed journal article
Supervised learning for arrival time estimations in restaurant meal delivery
Hildebrandt, Florentin; Ulmer, Marlin Wolf
In: Transportation science - Hanover, Md. : INFORMS . - 2021 [Online first]
Current projects
Meal-Delivery Operations
Duration: 01.01.2023 to 31.10.2025
We analyze planning and operations in restaurant meal-delivery, We consider the design of different delivery systems. We further optimize demand and fleet control in an integrated manner, and use machine learning for delivery time predictions.
Completed projects
Integrating machine learning in combinatorial dynamic optimization for urban transportation services
Duration: 01.09.2022 to 31.08.2024
The goal of this project is to provide effective decision support for stochastic dynamic pickup and delivery problems by combining the strengths of mixed-integer linear programming (MILP) and reinforcement learning (RL).Stochastic dynamic pickup-and-delivery problems play an increasingly important role in urban logistics. They are characterized by the often time-critical transport of wares or passengers in the city. Common examples are same-day delivery, ridesharing, and restaurant meal delivery. The mentioned problems have in common that a sequence of decision problems with future uncertainty must be solved in every decision step where the full value of a decision reveals only later in the service horizon. Searching the combinatorial decision space of the subproblems for efficient and feasible tours is a complex task of solving a MILP. This complexity is now multiplied by the challenge of evaluating such decision with respect to their effectiveness given future dynamism and uncertainty; an ideal case for RL. Both are crucial to fully meet operational requirements. Therefore, a direct combination of both methods is needed. Yet, a seamless integration has not been established due to different reasons and is the aim of this research project. We suggest using RL to manipulate the MILP itself to derive not only efficient but also effective decisions. This manipulation may change the objective function or the constraints. Incentive or penalty terms can be added to the objective function to enforce or prohibit the selection of certain decisions. Alternatively, the constraints may be adapted to reserve fleet-resources.The challenge is to decide where and how the manipulation takes place. SDPDPs have constraints with respect to routing, vehicle capacities, or time windows. Some constraints may be irrelevant for the fleet’s flexibility while others might be binding. The first part of the research project focuses on identifying the "interesting” parts of the MILP via (un-)supervised learning. Once the "interesting” parts are identified, the second challenge is to find the right parametrization. Here, we will apply RL methods to learn the state-dependent manipulation of the MILP components.
Opportunities for Machine Learning in Urban Logistics
Duration: 01.03.2020 to 31.08.2024
There has been a paradigm-shift in urban logistic services in the last years; global interconnectedness, urbanization, ubiquitous information streams, and increased service-orientation raise the need for anticipatory real-time decision making. A striking example are logistic service providers: Service promises, like same-day or restaurant meal delivery, dial-a-ride, and emergency repair, force logistic service providers to anticipate future demand, adjust to real-time traffic information, or even incorporate unknown crowdsourced drivers to fulfill customer expectations. Data-driven, anticipatory approaches are required to overcome the challenges of such services. They promise to improve customer satisfaction through accurate predictions (e.g., via supervised learning), enhanced fleet control (e.g., via reinforcement learning), and identification of demand patterns and delivery scenarios (e.g., via unsupervised learning). Within this research project, we combine recent advances in machine learning with established methods from operations research to tackle present-day challenges in urban logistics.
- Transportation Science Meritorious Service Award 2024
- Transportation Science Meritorious Service Award 2023
- Transportation Science Meritorious Service Award 2022